
In a previous discussion, we introduced the Green View Index (GVI), an index derived from satellite imagery. The GVI offers a "bird's eye" perspective on greenery within an area, calculated based on a resolution of 30m by 30m. This means that within each 30m x 30m grid cell, various elements such as trees and man-made structures (e.g., roads, buildings) are detected by optical sensors and aggregated into a single index. As a result, finer-scale objects are inherently generalized in this metric.
In our last analysis, we compared the Green View Index (GVI) directly with rent prices on a 1:1 basis, and the results were contrary to our expectations. Several factors could explain this outcome, including the multifaceted nature of rent determinants, the higher concentration of green spaces in suburban rather than urban areas, and the fact that densely populated urban zones tend to command higher rent prices.
Upon further consideration, one overlooked aspect could be that rent prices often reflect an area's quality of life. Satellite-derived green views might not necessarily correlate with the type of green spaces valued by residents.
To address this, we are now focusing on measuring greenery from a human-centric perspective, observing it from the street level. In this analysis, we will use Google Street View images and extract greenery estimates through computer vision methods. While scaling this analysis across wide regions is challenging due to the intensive computational requirements of processing Street View images, we will instead analyze a select number of census tracts using random sampling.
We obtain 360-degree panoramic images from Google Street View and apply cubemap projection to transform these into single images that capture all visible objects from the camera point on the street. Using semantic segmentation, a computer vision model, we then isolate vegetation from other objects in the imagery. The time frame for this analysis is limited to months when greenery is most visible, from May to October.


Figure: Cube-projected Google Street View
When input into a computer vision model, the system identifies and defines pixels classified as vegetation (excluding pixels at the bottom of the image, which correspond to the camera's base).


Figure: categorized street view image (green: green, blue: car, yellow: sidewalk, brown: building). The bottom image is merged image between original image and categorized image.
Due to the limitations of large-scale analysis with Google Street View (GSV) data, we conducted tests on a sample of 41 tracts within the NYC area, analyzing a total of 2,943 GSV locations.

The following images illustrate sample results, comparing locations with low green view versus those with high green view.
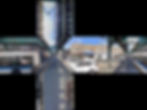
Figure: No green view
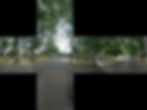
Figure: Sample location with the highest green view.
We calculated this for each individual view to analyze the proportion of green view occupying the visible area.

Figure: The diameter represents the magnitude of the green view value.
We averaged the green view values for each tract and compared them with rent per square foot. The results indicate that in Manhattan, rent per square foot is significantly higher than what the average green view of each tract would suggest. Excluding Manhattan, the scatterplot suggests a somewhat linear relationship between green view and rent in Queens and Brooklyn (Kings). In the overall sample regression model, however, the p-value was 0.74, indicating a lack of statistical significance.

When analyzing only Queens and Kings (Brooklyn), excluding Manhattan, a significant relationship was found between rent per square foot and green view, with a slope of 7.79 and a p-value of 0.01.

Conclusion
It is interesting to note the contrast between this analysis and our previous study, which used satellite imagery to calculate a vegetation index and found no significant correlation with rent prices. This suggests that rent prices may be more influenced by perceptional aspects of greenery experienced at the pedestrian level rather than the generalized vegetation index observed from satellite images. Although this small-scale analysis does not provide conclusive results, our prior satellite image analysis applied regional filtering and still showed no statistical correlation between rent prices and the satellite-based green view index. Therefore, a large-scale analysis might yield results somewhat aligned with our current findings.